As a followup to part one of his guide to computational creativity for those in creative fields, Thomas Euler looks at the current state of artificial intelligence today, and what sort of role it can and will play in the creation of music, writing and film.
_____________________________
Guest post by Thomas Euler of Attention Econo.me
This series is part two of my guide through the field of computational creativity for practitioners and executives in the creative industries. Here is part one, where I gave an introduction to the field.
Today, we’ll be looking at the current-state-of-the-AI in three creative domains: music, writing and video/movies. Keen observers will note that I talked about six domains in the introduction. True. Yet, I was overly optimistic in thinking I could cover all six in a single piece (I mean, sure, I could. In a 4,000+ word piece aka a 20+ minute read; those don’t work particularly well on the web though). Thus, I changed the format on the fly. It’s now a five-part-series. I’ll cover painting, games, and advertising next week.
Music
The idea that algorithms can write music has become commonplace over roughly the last three years (though the field goes back to the 1950s). Last week, I mentioned Daddy’s Car. It’s a Beatles-style Song which Sony’s AI research team released last year. The song was composed by the AI system FlowMachines and, then, arranged and recorded by humans. The technology at work was a machine learning algorithm that was trained on old Beatles songs. It extrapolated some typical characteristics of the band’s music — in the machine’s logic some statistical properties like what note usually follows another and similar patterns — and wrote a new piece based on its assumptions about what a Beatles song is (it didn’t write the lyrics).
This exact approach is behind most of the AI music that recently came to the public’s attention. It’s noteworthy that it no longer comes primarily from the scientific community. Instead, there are several startups and established companies getting into the field. Which is a good indicator of a technology’s increasing maturity: at least some people believe that it has reached the point of commercial viability.
Two notable startups in the field are Aiva and Jukedeck (both from Europe, btw). Their approaches are pretty similar, at least from a top-level perspective: both use generative systems that create music. The key difference is how the music is actually made. Jukedeck has its software produce the tracks while Aiva uses human musicians to record the AIs composition. As a result, their respective business models and target markets differ.
Jukedeck runs a self-service platform that allows users to create tracks based on a few simple input parameters like genre and mood. It has low prices (royalty-free licenses cost $0.99 for individuals and small businesses, $21.99 for large businesses; the full copyright costs $199) and minimal customization options. As such, it is an interesting option primarily for online creators that need music (e.g. podcasters or YouTubers) as well as digital content teams of bigger brands.
[soundcloud url=”https://api.soundcloud.com/tracks/306367994″ params=”color=ff5500″ width=”100%” height=”166″ iframe=”true” /]
Aiva, in comparison, positions itself more as an upmarket solution. Its system — which is the first AI registered with an author’s rights society — produces music on request. The company’s website describes that it composes “memorable and emotional soundtracks to support your storytelling”. In a recent interview, the founders are more explicit about the market they want to serve: buyers of soundtracks for movies, games, commercials, and trailers. Those, of course, rely on more customization and higher production quality.
[soundcloud url=”https://api.soundcloud.com/tracks/284587399″ params=”color=ff5500″ width=”100%” height=”166″ iframe=”true” /]
Still, the structural similarities between both companies are obvious. Neither thinks its business is selling artistic output to fans (though you can buy an album from Aiva). Instead, they address a class of buyers who use music as a supportive element within their multimedia creations.
Google, one of the big and leading players in artificial intelligence, is also doing some work around music. Although, I should say, in a way more experimental fashion (the luxury of being free from immediate financial pressures). The company doesn’t currently monetize any of its efforts. These center around Magenta, a project by the famous Google Brain team that leads Google’s machine and deep learning research.
Magenta is interesting because it’s going in a different direction than the aforementioned startups. While its long-term goal, too, is to build algorithms that can create music (and art) on their own, the current releases are more narrow in scope. They have built a Neural Synthesizer (which does some pretty interesting stuff) and a note sequence generation model that responds to a human musician. There are standalone demos of both online. More interestingly, though, Google also provides plugins for Ableton, one of the leading digital audio workstations. That is, they are building tools for musicians (of course, powered by Google’s open source machine learning library Tensorflow).
The philosophical difference to Jukedeck and Aiva is obvious: While the former’s technology is the creator, Google’s approach is to build tools that creators can use or collaborate with. Judging by Douglas Eck’s presentation at this year’s Google I/O developer conference, the idea of AI as a tool and collaborator is firmly ingrained in Magenta’s strategy. That said, the team is also working on recurrent neural networks which create music (this postnicely summarizes some of the challenges involved).
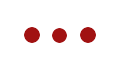
Writing
One of the critical things to understand about today’s AI systems lies in the difference between syntax and semantics. Algorithms have become quite good at syntax; that is, understanding the rules of language and how we construct sentences. As I explained in part one of this series, the modern machine learning algorithms are based on statistics. Their output is probabilistic: what word is most likely to occur after this word, what phrase after that phrase?
So, syntax is right in the domain of today’s AI. But semantics, understanding the meaning of words, is an entirely different animal. While there used to be a lot of buzz about semantic search a few years ago, even those systems relied on improved word counting.
When humans use language, the words we choose are representations of concepts and ideas we have in our minds. Many of our words are associated with emotions. We intuitively use and understand metaphors, proverbs or analogies; for computers, these constructs are very hard. Why? Because the regular meaning of the words we are using isn’t what we are talking about in these instances. An old but still prevalent problem in the field of natural language understanding.
What so-called natural language generation (NLG) algorithms can do, is use words to create grammatically correct sentences. Some even have an extensive database of words that are often associated with other words, of synonyms, and related concepts. But no AI understands the meaning of those words like humans do. After all, computers deal with language in a binary fashion. Humans don’t.
This, naturally, is a limitation when it comes to writing. Still, automated writing has made some progress over the last few years which resulted in useful applications. Don’t expect algorithms to write proper novels or think pieces anytime soon (not even in Japan). But where software excels at, is data-based writing and scaling text.
The Washington Post — since being acquired by Jeff Bezos one of the more tech-savvy publishers out there — has developed its own system Heliograf. On last year’s election day in the USA, for instance, they used it to cover 500 local elections (semi-)automated by creating stories from publicly available data. In this article, the Post explained Heliograf in more detail:
“We have transformed Heliograf into a hybrid content management system that relies on machines and humans, distinguishing it from other technologies currently in use. This dual-touch capability allows The Post to create stories that are better than any automated system but more constantly updated than any human-written story could be,” said Jeremy Gilbert, director of strategic initiatives at The Post.
Using Heliograf’s editing tool, Post editors can add reporting, analysis and color to stories alongside the bot-written text. Editors can also overwrite the bot text if needed. The articles will be “living stories,” first beginning as a preview of a race in the days leading up to the election. On Election Day, stories will update with results in real-time and then, after a race is called, the story will provide analysis of the final results. Geo-targeted content will surface in The Post’s liveblog and in special Election Day newsletters to readers, offering updates on races in their state. Internally, Heliograf also surfaces leads for potential stories, and on Election night, will alert reporters via Slack when incumbents are lagging and races are called.
Similar tools are offered by Arria, Automated Insights, and Narrative Science. These companies sell different NLG solutions that turn data into text. The sophistication of the underlying technology differs — Automated Insight’s Wordsmith, for example, is largely based on pre-written templates — but the general idea is the same: The software is fed with (structured) data and creates text from it.
Use cases include financial reports, sports game summaries or management reports. Looking at the client lists of those companies, it’s apparent that the majority of customers are businesses that use the software to create reports from their data. Another area where the technology is useful is creating and maintaining highly standardizable content (e.g. product information or player data) on large portals or shops.
Videos & Movies
Most videos and movies involve humans. They are the actors/protagonists, cameramen and -women, and handle many other tasks in the production process. As a result, an autonomous AI filmmaker that just spits out ready-made movies is even further away than in other creative disciplines.
However, that doesn’t mean there’s no place for AI in the video industry. There are several applications for artificial intelligence along the creation process. As this series focuses on computational creativity, let’s look at the most obvious creative step: script writing. If you’ve been following the subject of computational creativity last year, you might have come across two pieces of news which involved the words “AI”, “movie”, and “written”. Of course, all the problems I pointed out in the writing section also apply to movie scripts.
Yet, in June 2016, the short film Sunspring debuted online. The script was written entirely by a recurrent neural network (that named itself Benjamin). Humans, then, took the script and made the movie for the 48-Hour Film Challenge of the Sci-Fi London film festival. The result is definitely worth watching, particularly because the cast made the most out of the writing. To call the latter abstract would be an overstatement. A Leos Carax movie looks easily accessible in comparison.
A few months later, a Kickstarter campaign for a horror movie called Impossible Things gained some viral exposure. The campaign successfully funded the production of “the world’s first machine co-written feature film” (it’s set to release later this year). The “co” bit in there is important. The algorithm that co-wrote the script was effectively trained on plot summaries and financial performance data of horror movies, from which it then inferred a series of popular plot points. The actual writing was performed by a human.
So, the attention both cases received has more to do with the inclusion of “AI” in the press releases’ headlines than the quality of the writing. It’s the other end of the production process, where AI applications look more promising right now: in editing.
IBM made some headlines last year when Watson “made” the trailer for the movie Morgan. This, too, was a promotional stunt. Watson didn’t make the trailer, at least not entirely. What happened was that it was given a bunch of trailers to analyze. Based on this input, it suggested scenes from Morgan that corresponded with its idea of a trailer. The trailer’s making-of contains a scene that depicts Watson’s output:
This, then, was used by a human editor to cut the actual trailer. Don’t misunderstand me: this isn’t nothing. If algorithms can reliably sort through hours of material, it’s most certainly a welcome help to editors. In this regard, the recent progress made in object recognition in videos — particularly by Google — is noteworthy as well. Imagine a documentary maker’s editing software could go through 100+ hours of footage in a few minutes and find all scenes containing x. This isn’t far off anymore.
Some consumer apps go even further. They allow users to upload their clips and the software automatically generates a movie from it. Examples are Shred, which specializes in GoPro-style sports and adventure footage, or the general purpose Magistro. Both offer mobile apps and desktop solutions and are focused on consumers who make video on their smartphones but don’t want to edit their footage. While the resulting videos aren’t up to professional standards, the services do a surprisingly decent job and are likely good enough for many users.
That’s it for part two. Next week, we’ll look at the current state-of-the-AI in the fine arts, computer games, and advertising. Make sure to subscribe to the newsletter below, so you don’t miss it.
Thomas Euler is an analyst, writer. At the intersection of tech, media and the digital economy. Founder of www.attentionecono.me. For more info check: www.thomaseuler.de | Munich